The tragedy of commons in OSS
A data essay exploring symptoms, remedies, and opportunities Recently GitHub released For Good First Issue, a special index that tracks Digital Public Goods (DPGs) repos. This includes open-source software, open data, open AI system, or open content collection that supports the United Nations sustainable Development Goals (SDGs). Currently, the official registry counts ~132 projects which includes a variety of social and impact-focused projects like a webapp to track hypertensive patients across a population, an app for citizen participation and open government, and software to help social workers manage data on vulnerable children. For Good First Issue addresses a significant need in the open-source ecosystem: directing dedicated and socially conscious talent to projects that positively impact society and would otherwise struggle to attract contributors. Initiatives like this one can help raise the collective consciousness of contributors and ameliorate the negative externalities that arise from the tragedy of the commons. The tragedy of the commons In economics, the tragedy of the commons refers to the situation in which individuals with access to a public resource act in their own interest and, in doing so, ultimately deplete the resource. Though this concept is traditionally associated with shared environmental resources, it can also extend to the domain of open source software. While resources canât be depleted in the context of software, they can be regarded as abused when users do not donate time, work, or resources to the communities they take from. In these situations one can observe a number of negative consequences like slow development, weakened community support, or even legal and licensing risks. The famous monetisation and maintainer problems in open source are nothing less than a manifestation of this economic pathology. A number of initiatives have tried to ameliorate the tragedy, but no definite solution exists yet. In light of GitHubâs recent initiative it is important to understand in what ways the tragedy of the commons manifests in open source, and whether curated contributor indices like DPGs can help break it. In particular, we investigate whether initiatives like DPG projects present a real opportunity to current or aspiring open source contributors. To do this, we compare the set of DPG projects on GitHub against three other sets of open source repos, 2,395 projects from the Apache foundation, 8,129 projects under the Linux Foundation umbrella, 6,805 projects maintained by RedHat. 1,255 repos belonging to Digital Public Goods, To perform this comparison we derive traction and engagement metrics using publicly available GitHub data. Specifically, we look at three fundamental metrics: issue backlog, contributor retention, and maintainer responsiveness. These metrics will help us understand the overall health of each group of projects, as measured by the activity metrics that most strongly correlate either with the resources available to a project or with the communityâs support to the project. This will help us understand whether DPGs are particularly affected by the tragedy of the commons and the type of developer experience (DX) that new contributors can expect. #1: DPGs have (on average) less contributors per issue than other groups An important indicator of the âtragedy of the commonsâ in open source is the size of the of the issue backlog a project has. In other words, the number of unresolved open issues in the repository. Although not every open issue represents critical technical debt, an imbalance between issue creations and number of merged PRs suggests a lack of resources to manage them effectively. Public data shows that DPGs have the highest concentration of open issues per repository, but also the fewest number of contributors per issue. While this indicates a scarcity of contributor resources, the relative high number of open issues also suggests an opportunity for contributors who want to make a significant impact by applying their software skills to for-good projects. #2: DPGs have less long-term contributor retention than RedHat & Apache Contributor retention is important to keep a projectâs issue backlog under control. To better understand contributor engagement we compute âOn or After Contributor Retentionâ which we define as the percentage of contributors who merge a Pull Request (PR) at a specific time or any time thereafter after the first contribution. Interestingly, DPGs show the highest âOn or After Contributor Retentionâ contributor within a three month period. However, this engagement drops significantly after month 3 and stays below the retention shown by Apacheâs and RedHatâs projects. Itâs worth noting that DPGs have the hightest short-term retention, but that six months after the first contribution 60% of them donât contribute again. #3: DPG maintainers are as responsive as maintainers from RedHat and the Linux Foundation. Another strong indicator of resource scarcity in a project is the waiting time a contributor experiences after submitting a PR, specifically, the average time they wait to see their PR merged into the codebase. If a maintainer has more bandwidth, they can review and give feedback more quickly, thus decreasing the average merge time. Notably, public data shows that DPG repositories exhibit a level of maintainer responsiveness comparable to that of RedHat and the Linux Foundation and superior to that of the Apache Foundation. This indicates that the level of Developer Experience (DX) when contributing to these repos is comparable to that of more established and popular projects. Breaking the tragedy of the commons Letâs get contributing! Our analysis shows that DPG repos are rich in open issues and that in some respects (like maintainer responsiveness) one can expect a similar level of DX than repos under the RedHat or the Linux Foundation patronage. As the number of DPG projects grow, itâs important for aspiring contributors to use an index to browse for the most relevant communities. Considering that open source is the most secure model for developing AI systems for the public, we anticipate a significant growth in DPGs indices over the next few years. GitHubâs For Good First Issue maintains a special index of âFor Goodâ repos, while platforms like Quira index repos across the ecosystem and helps you discover and filter issues that align to your language and topic preferences. In fact, we just released a new interface that allows you to not only select DPG repos, but also repos in other categories mentioned in this essay like the Linux Foundation. Try it out đ However, to fully break the tragedy of the commons itâs also important to make sure that projects are well funded and that part of those funds are distributed equally to core maintainers and casual contributors. Funding a project is now straightforward thanks to products like GitHub Sponsors, BuyMeACoffee and Patreon. However, distributing funds to casual contributors is less common, but platforms like Quira are launching ML-powered products that enable organisations to pay their contributors when they merge meaningul and valuable PRs. This can help organisations retain contributors and encourage them to return to contribute in situations where the opportunity cost of contributing is high for the developer. Do you have an open source project and would like to create a reward scheme for your contributors? Get in touch!đą Thanks to Alex Bird for his help in preparing this essay and to Kasiasun for her feedback and comments â Artwork from asciiart.eu â Written with â¤ď¸ by Quira
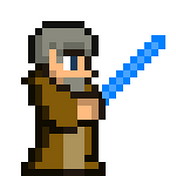
By Rodrigo Mendoza-Smith
â˘
Mar 21, 2024
â˘
9 min read
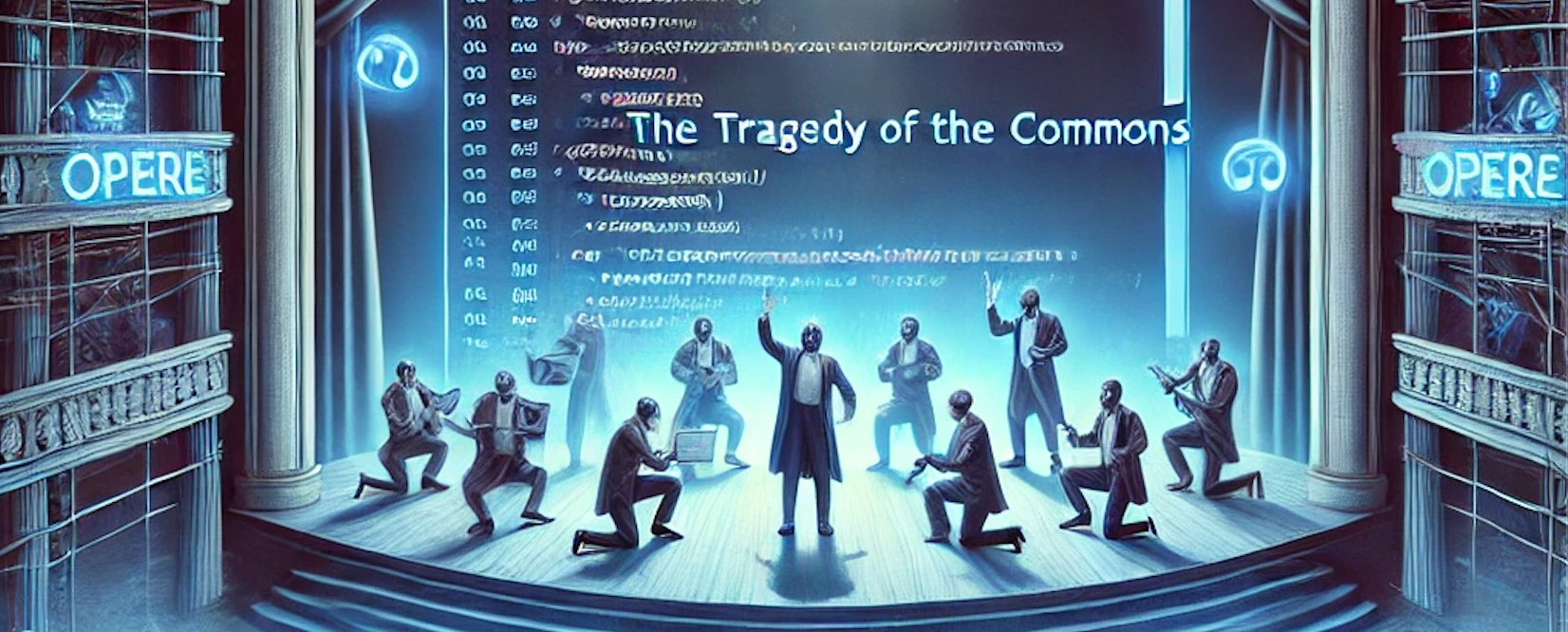